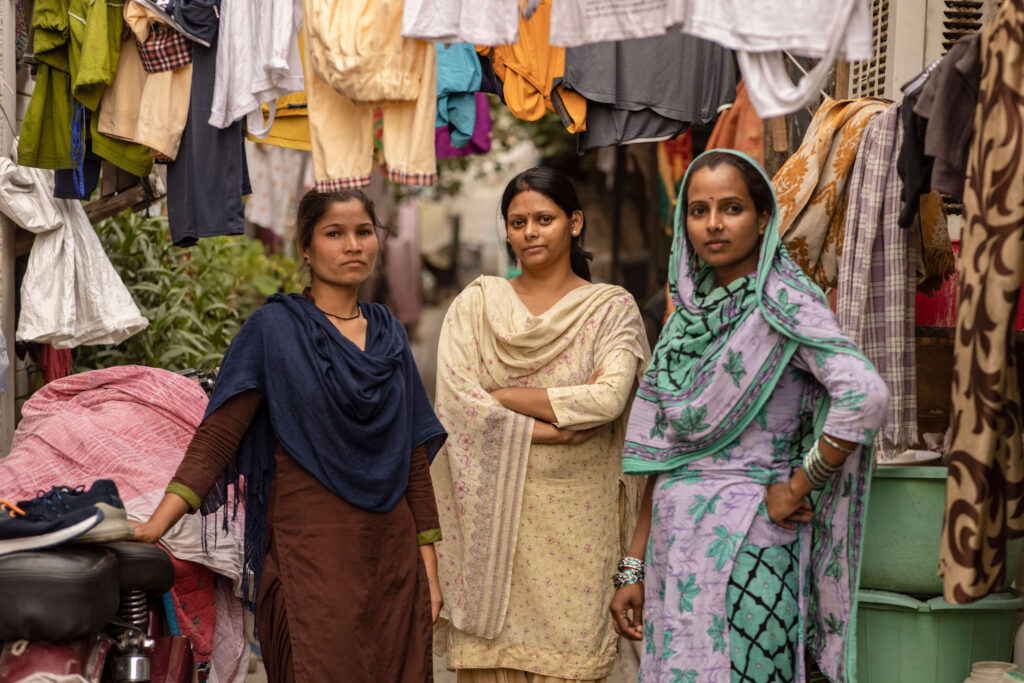
“Essentially all models are wrong, but some are useful,” British statistician George E. P. Box declared. Despite progress made in machine learning and artificial intelligence, digital credit models risk being considered “wrong, but useful” – useful for certain groups such as urban salaried workers, but wrong as they leave behind large segments of the economy whose data trails are too faint or complex for a credit scoring algorithm.
CFI recently explored how automated decision-making tools for digital credit are playing out for one demographic in India – the more than 54 million interstate migrants – and what this might teach us about the ways data inputs and algorithms intersect with the digital lives of those at the margins.
Digital Credit in India is Booming, Yet Leaving Behind Large (and Possibly Viable) Segments of the Economy
The fintech ecosystem has ballooned in India in recent years, with an estimated 2,000 fintech startups and 300 digital lenders, including Buy-Now-Pay-Later (BNPL) players, operating by the end of 2020. Digital lending alone is expected to reach $1 trillion by 2023. While digital lenders existed prior to the pandemic, they have boomed over the last two years in the rush to conduct business and daily tasks remotely.
Earlier this year, CFI conducted desk research and interviews across the digital finance ecosystem in India and learned that most digital lenders are focused on serving urban, young, and employed consumers. With higher and more predictable income streams, this customer segment creates large volumes of digital data that can be mined to determine creditworthiness. And given the overall size of the Indian market, there is still room to grow before even this segment is saturated. But where does that leave the rest of consumers, including migrants?
While migrants have propelled the growth of vital industries such as construction, hospitality, and commercial agriculture, they historically have faced hurdles in accessing formal financial services and government benefits. And now with the increasing reliance on digital data to allocate benefits and make credit decisions, migrants potentially could be further marginalized.
A migrant’s data trails do not fit the mold built for the urban, young, employed segment digital lenders were designed to serve.
Take the case of Mohan Kumar, an archetypal migrant whose data trails systematically exclude him from financial services. Mohan works in Surat, India in a garment factory, but is originally from a village near Ganjam, Odisha where his family still lives. Recently married, he visits his wife, who now lives with his parents back in Ganjam, for two weeks twice a year. On his last visit home, Mohan and his wife decided to open a stationary store. When seeking advice from friends, Mohan learned about digital loans available through the phone. Although small, these loans helped a few of his peers with fledgling, entrepreneurial ventures. Despite having a steady, full-time job in Surat for the last four years, Mohan is rejected again and again from numerous digital lenders. Simply put, his data trails do not fit the mold built for the urban, young, employed segment they were designed to serve.
Millions of migrants face similar challenges as Mohan. In general, migrants have fainter data trails due to lower levels of digital activity. Many low-income migrants have limited financial and digital capability and do not possess smartphones. And those who do have a smartphone typically only use mobile data when necessary due to the high cost. Additionally, migrants often rely on agents, such as kirana store owners, to assist them with digital transactions, and these transactions may not be directly associated with their identity.
Furthermore, digital lending algorithms do not understand the data trails of migrants very well. Migrants’ mobility causes multiple barriers. Some migrants change phones and phone numbers between their destination and home, which can impede the verification of transactions or authorizing identity via one-time-passwords (OTP), leading to KYC failures. Additionally, a mismatch between the location of the cell tower where a potential borrower applies for a loan and the location where the phone’s SIM is registered could cause the applicant to be rejected. While providers rationalize that the geographic mismatch might imply that a customer would be hard to reliably reach, unfortunately, this can unintentionally screen out viable borrowers who are inherently on the move, such as migrants.
Migrants’ payment patterns are not well-understood or formalized, organized, or digitized.
When it comes to assessing cashflows to determine repayment capacity, migrants’ digital data trails make it difficult to see the full picture of their economic lives. For example, even if a migrant has established a stable income over multiple years, as is the case with Mohan, often their payment patterns are not well-understood or formalized, organized, or digitized. For example, some contractors provide an advance payment while others pay weekly or monthly, and some cover living expenses for migrants but deduct the costs from their salaries. In addition, migrants’ data trails might show varied consumption patterns in their two locations – for instance, in Surat, Mohan spends his money on accommodations and food, while back in Odisha he spends on household expenses and children’s education. Such variations in earning and spending tend not to fit within lending models’ range of what makes a viable borrower.
Finally, there is the issue of accessibility. Examining the top 30 downloaded digital credit apps in India (15 on the App Store and 15 on the Google Play Store), we found that 28 out of 30 worked only in English, with just 2 offering Hindi. Such limitations favor highly educated segments and inherently exclude vast numbers of potential clients.
More Effort is Needed to Ensure Digital Credit Does Not Further Exclude Marginalized Groups
The Reserve Bank of India (RBI) has taken an interest in digital lending and consumer protection issues over the last two years, asking Google to remove at least 30 lending apps from the Play store that did not comply with local laws, convening a working group under the chairmanship of executive director Jayant Kumar Dash, and empowering FACE to become a self-regulatory organization (SRO). The working group focused on a range of consumer protection issues including transparency and disclosure, aggressive sales, consent, and algorithmic design. For instance, one of the group’s recommendations suggests that providers have verifiable audit trails for their algorithms and models should be documented to ensure transparency. While a promising direction, little is understood about what this will look like in practice and how it will balance accountability with the proprietary nature of the models.
Moving forward, digital lenders should find ways to understand and assess the unique activities of groups like migrants through more nuanced models and data inputs that give a more complete picture of a migrant’s economic life. For example, do workers travel back to the same destination each working cycle? How many trips home do they make? How have income patterns shifted within their employment – is it consistent or is it varied?
Digital lenders should find ways to understand and assess the unique activities of groups like migrants through more nuanced models and data inputs that give a more complete picture of a migrant’s economic life.
Providers could draw inspiration from the work of India Migration Now (IMN), which is working to understand the nuances of the data trails of migrants. They are preparing to launch a pilot for a customized, digital lending product for migrants that will leverage both traditional and non-traditional indicators to determine creditworthiness. IMN’s scoring model will leverage data inputs such as frequency of travel, place of origin, destination for migration, frequency of remittance transfer, length of stay at migration location, GPS, and mobile top-up amounts. IMN’s focus on migrants requires commitment, research, time, and resources, which may be less attractive to other digital lenders when there are still potential customers with more robust data trails who are easier to reach.
What Can Be Done to Maximize the Inclusivity of Algorithms?
Research: We must do more to understand whether specific segments that are already at risk for exclusion are further included or excluded by algorithmic decisions. Researchers could help by leveraging context-specific research about specific segments – for instance, migrants or scheduled tribes in India – to help data scientists better understand the data trails of these groups and incorporate insights into models.
Guidance and Incentives for Providers: While advancements in auditing and disparate impact testing can measure models’ results across a set of sensitive characteristics (e.g. gender, race, religion, ethnic group), providers may not prioritize the inclusivity of their algorithms. In markets like India, for instance, financial service providers continue to focus on growth among customer segments with more “intuitive” data trails and on keeping customer acquisition costs low. In our view, stakeholders such as impact investors and industry associations can engage with providers to provide guidance and/or incentives for inclusive algorithms. CFI recently launched a project to help impact investors engage with fintechs on their algorithms during their due diligence processes.
Government Engagement: Because this is a very nascent field, more work is needed to test regulatory approaches and efforts to build the public sector’s capacity for supervision. Government entities, such as the Reserve Bank of India, have begun to think more strategically about the supervision of high-stakes algorithmic decisions. Ongoing work is needed to understand and advance data protection regulation, national AI strategies, and sector-specific initiatives, such as the RBI working group or Project Veritas in Singapore, to name a few.