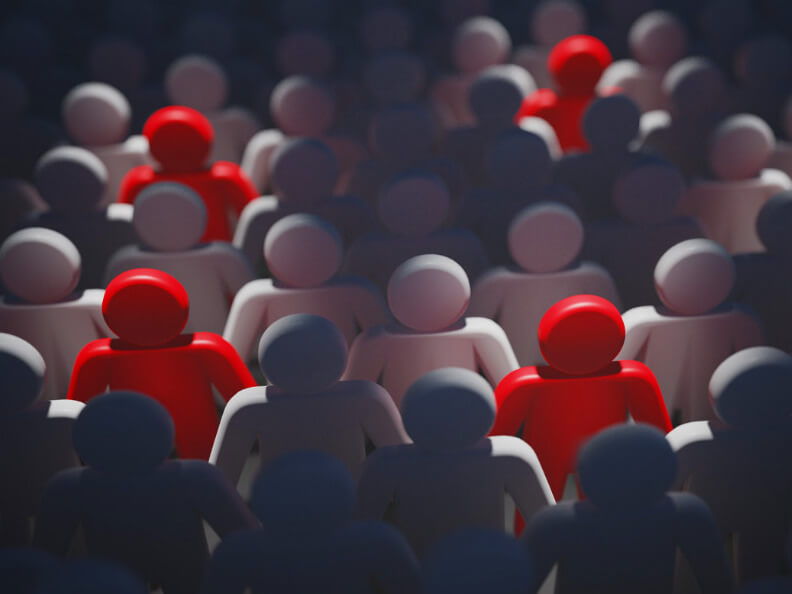
When Goldman Sachs came under fire for offering women lower lines of credit than men on the now infamous Apple Card, they defended the product against charges of sexism by explaining that gender had not been an input to the credit scoring model. If an applicant’s gender was never fed into the algorithm, how did credit decisions end up discriminating against women who had similar credit histories as men?
Gender-blind credit scoring is an example of what many AI bias researchers call “fairness through unawareness,” an approach that intentionally excludes data on sensitive attributes like race, ethnicity, religion, or sex with the goal of reducing discriminatory outcomes. Behind this approach is the idea that eliminating markers of different group identities will prevent basing decisions on those characteristics. This has led to failures in the past, notably redlining. Recent research has shown it can also make discrimination harder to detect and address in the era of algorithmic decisions.
“Fairness through unawareness” intentionally excludes data on sensitive attributes like race, ethnicity, religion, or sex with the goal of reducing discriminatory outcomes.
As algorithms transform the financial sector, we need a better understanding of how to mitigate against discriminatory outcomes to ensure that those left out of the digital ecosystem today do not face further financial exclusion. Otherwise, we may not know how many Apple Card equivalents exist in inclusive finance.
This post explores the challenges and tradeoffs of using sensitive attribute data for detecting discrimination in the context of evolving data protection norms and regulations.
The Perils of Fairness through Unawareness
The idea may seem counterintuitive: if you remove gender from the data inputs of a credit model, how does the algorithm consistently assign a lower limit to women? The reason is that credit scoring algorithms are crunching larger amounts and new sources of data, which means that the model can inadvertently use proxies for sensitive attribute data in decisions, even if that was not the intention of the model developers. For instance, the type of phone someone uses or the apps a person installs on their phone can act as proxies for gender, age, or other characteristics.
Models can inadvertently use proxies for sensitive attribute data in decisions, even if that was not the intention.
A very simple method for testing algorithmic bias within a credit scoring model requires only a few variables like the actual default rate, the actual score of the individual when approved, and the variable you want to test with your algorithm, such as gender or race.
Data Tensions: Privacy vs. Anti-Discrimination
So, why isn’t this done? The answer lies in data limitations, sometimes based on very legitimate concerns about histories of injustice. It illustrates one of the challenges of detecting and monitoring for bias in algorithmic systems without infringing on privacy rights.
Many countries around the world prohibit collecting sensitive data or basing credit decisions on protected categories either under their banking regulations or under new data privacy legislation. In India, lenders are prohibited from discriminating on the grounds of sex, caste, or religion. In the U.S., the Equal Credit Opportunity Act (ECOA) made it illegal for credit providers to discriminate based on sex, race, national origin, and other protected characteristics in response to widespread denials of credit to women and people of color. ECOA prohibits lenders from collecting this data in the first place, even for the purpose of detecting discrimination, because of fears it will be used improperly to further perpetuate bias in consumer lending. Research in the US shows that discriminatory trends have been hard to detect in consumer lending and that a lack of sensitive attribute data has also created enforcement challenges.
While not all lending regulations prohibit the collection of sensitive data, data privacy legislation introduces new limits on this data. The General Data Protection Regulation (GDPR), which has become the model for legislation in many developing markets, bars certain sensitive data from being collected or processed — including data on race and ethnic origin, political opinions, philosophical beliefs, sexual orientation, and biometrics — without individual consent.
Concerns about safely and properly using sensitive attribute data are valid given histories of discrimination and surveillance of minority groups in both wealthy and developing countries.
On top of GDPR, the European Commission has recently put forward draft legislation to regulate artificial intelligence, with specific provisions for AI systems classified as high risk, which includes credit scoring. The draft legislation suggests that AI providers should process “special categories of personal data” to monitor and correct for bias in the public interest, but does not clarify collection practices or consent processes for such data. Concerns about safely and properly using sensitive attribute data are valid given histories of discrimination and surveillance of minority groups in both wealthy and developing countries. Changes in regulatory frameworks to allow for use of this data to mitigate against further discrimination require public trust bolstered by technical solutions that protect against misuse. Depending on a country’s history or in places experiencing active or simmering conflict, technical solutions may not be enough. For example, in a country like Rwanda where ethnic distinctions are banned, the tensions around sensitive identities make it difficult to fathom collecting and using such information, especially by the government.
Data Ecosystems in Emerging Markets: It’s Complicated
This tension between privacy and anti-discrimination may be exacerbated in developing markets by the limited digital footprints of customers at the data margins, as well as paltry knowledge on how data trails and protected groups intersect.
Sex-disaggregated data is still largely unavailable in the financial sector and for many economic indicators in developing markets. Beyond availability, there is less understanding of how women’s lives and the barriers that shape their economic behaviors are reflected in data used for credit decisions. The reality is that gender, ethnic, or religious identities interact with other indicators (like income, asset accumulation, or technology use) and will impact individuals’ data trails in ways that may misrepresent individuals’ potential and result in disparate outcomes (i.e. access to a financial product). Fairness through unawareness approaches are blind to this intersectionality.
Where Do We Go From Here?
As an industry, we can do more to understand the data inputs for digital financial services and how socioeconomic realities present in these datasets, especially for traditionally excluded groups. There will still be tradeoffs between privacy and anti-discrimination, but managing those tradeoffs requires a conversation between providers, regulators, donors, and the public to identify what is possible and explore the right balance.
These conversations can start with the many open questions that exist from a technical perspective. For instance, can third parties play a role in securely storing sensitive data, or are there options to use encryption or synthetic data techniques? Can regulators develop tools that allow them to estimate the demographic characteristics of a dataset, like the Consumer Financial Protection Bureau has done in the U.S.? Can investors encourage investees to design monitoring and evaluation indicators to help answer questions about how their algorithms perform on inclusion?
While the road ahead may be complex, starting these conversations and bringing the public into them, especially those whose lives will be affected by algorithmic decisions, will be crucial for building trust. We need to engage with emerging research on possible solutions to see what might be appropriate in developing markets. At the very least, financial inclusion stakeholders should know enough to respond to any gender-blind or fairness through unawareness approaches in digital finance with healthy skepticism.
Starting these conversations and bringing the public into them, especially those whose lives will be affected by algorithmic decisions, will be crucial for building trust.